Edtech startup Mappi wants to give everyone a personal AI math trainer! ENCCS helped Mappi with supercomputer access and we had the opportunity to chat with CEO & co-founder Karl Flintberg.
Can you tell us a bit about Mappi and how you do things differently?
Of course! Mappi is a math studying website for high school students with the goal of redefining Math Learning using Data and Generative AI. When working on Mappi we follow along students’ work, looking for strengths and blindspots, to generate tailored studying material and tutoring. Through this, we can avoid the traditional one-size-fits-all approach in math education!
What challenges did you face in developing your AI model before you had access to HPC resources?
When working with LLMs in math learning and education specifically the non-deterministic nature becomes a big problem. Fine-tuning a general model lets us bypass this problem. Doing that on a 70B parameter model on your own laptop is a no-go if you want to avoid frying your not-so-fine-tune-friendly GPU.
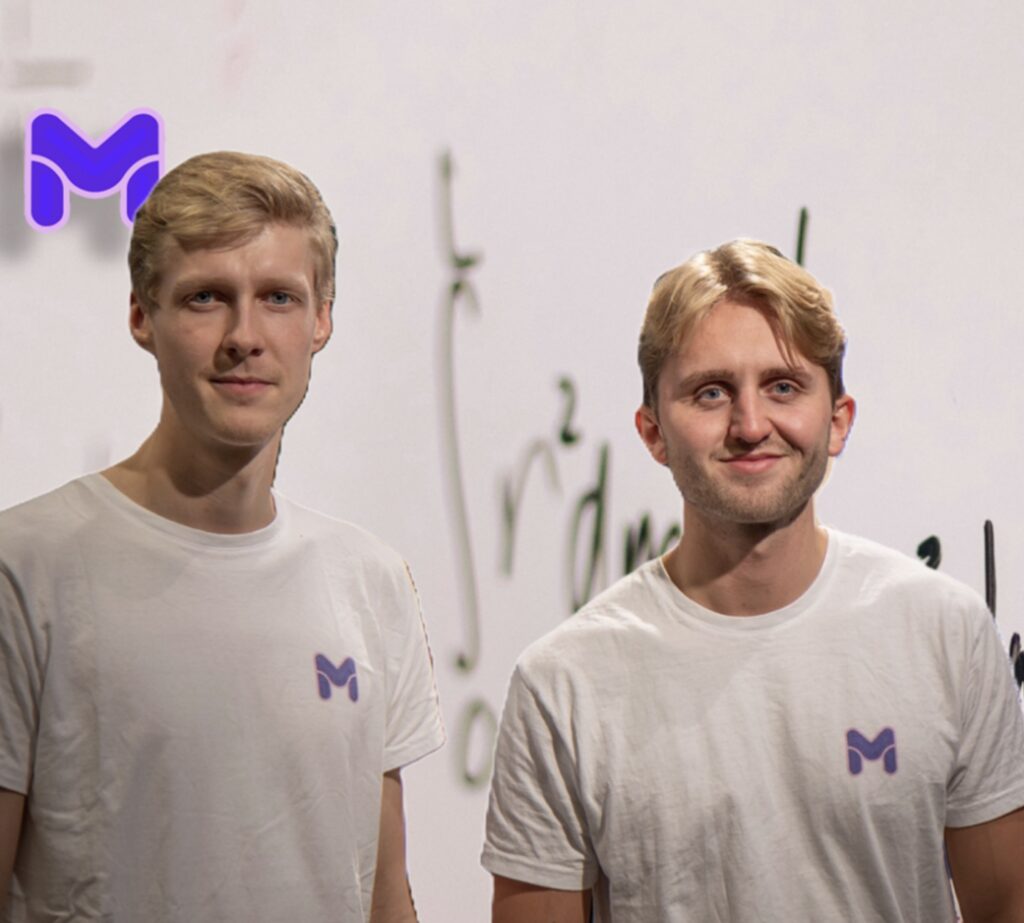
How has access to high-performance computing impacted your development process?
Specifically in the development process, the biggest difference is how we work towards more qualitative outputs. Before being able to access fine-tuning possibilities the path to more qualitative outputs is limited to primarily more advanced prompting. Having access to high-performance computing opens the door to a development process more focused on implementing specific fine-tuned models on specific learning tasks.
What advice would you give to other EdTech startups looking to leverage AI in education?
My best advice is to try out all three possible AI alignment approaches and keep in mind how they all have different purposes. Depending on your application all three techniques, prompting, RAG and fine-tuning, might not be necessary or one approach might not be enough to reach your criteria for qualitative output. Test and iterate!
What’s next for Mappi and your AI model?
The next step now is to test our fine-tuned models in production. Iterate on what works and what doesn´t, talking to our users about how they experience the effect of having math-specific AI models working to enhance their studying experience. We are looking forward to the possibilities that are opened through EuroHPC JU!
ENCCS helps you with getting access to supercompers
We help companies and organisations acquire access to supercomputing systems for your R&D. For more information please visit https://enccs.se/get-supercomputer-access/.